In a development that may enable a wholly new approach to artificial intelligence, researchers at Harvard University's School of Engineering and Applied Sciences (SEAS) have invented a type of transistor that can learn in ways similar to a neural synapse. Called a synaptic transistor, the new device self-optimizes its properties for the functions it has carried out in the past.
One of the more remarkable features of the human brain is it gets better at whatever it does. While your first day on an assembly line may be full of fumbling and confusion, in a week or two you will find yourself seemingly on autopilot, performing the set of required tasks without much mental effort. After a few months, you will respond automatically when a part comes through damaged or improperly oriented. Plasticity is the name for the brain's ability to change its own structure through thought and activity.
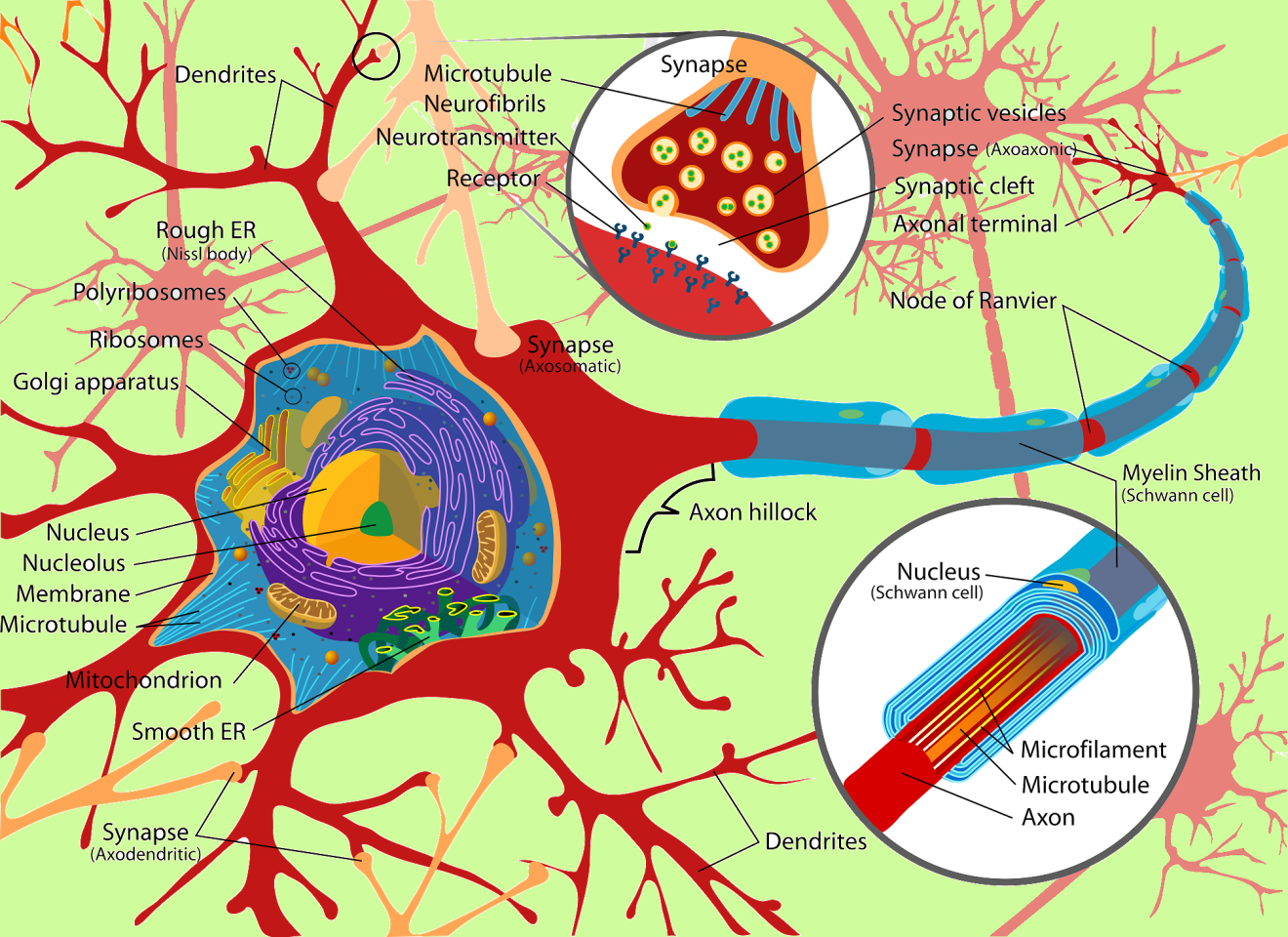
Most of this plasticity results from changes in the 100 trillion or so synapses, or interconnections, between brain cells. One of the ways through which sets of behaviors are reinforced, or learned, is called spike-timing dependent plasticity, or STDP.
Often summed up by the aphorism "Cells that fire together, wire together", when neuron A repeatedly sends a signal across a synapse that causes neuron B to fire, the synapse will strengthen, in effect making that decision easier to make in the future.
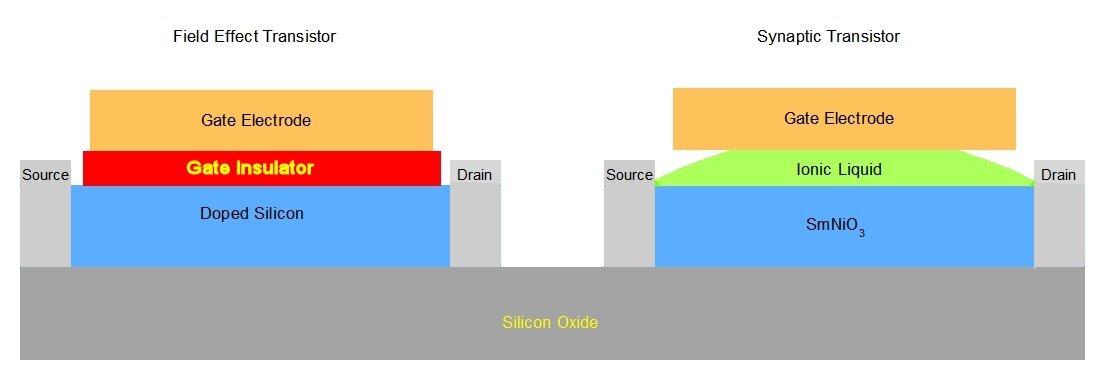
The synaptic transistor developed at Harvard mimics this behavior. So how does a synaptic transistor work? As shown above, the synaptic transistor has a structure quite similar to that of a field effect transistor, where a bit of ionic liquid takes the place of the gate insulating layer between the gate electrode and the conducting channel, and that channel is composed of samarium nickelate (SmNiO3, or SNO) rather than the field effect transistor's doped silicon.
A synaptic transistor has an immediate response, and also a much slower response related to learning. The immediate response is basically the same as that of a field effect transistor – the amount of current that passes between the source and drain contacts varies with the amount of voltage applied to the gate electrode. The learning response is that the conductivity of the SNO layer varies in response to the STDP history of the synaptic transistor, essentially by shuttling oxygen ions between the SNO and the ionic liquid.
The electrical analog of strengthening a synapse is to increase the conductivity of the SNO, which essentially increases the gain of the synaptic transistor. Similarly, weakening a synapse is analogous to decreasing the electrical conductivity of the SNO, thereby lowering the gain.
Note that the input and output of the synaptic transistor will be continuous analog values, rather than more restrictive digital on-off signals. This gives the artificial synapses the flexibility to learn "more or less" how to perform a task, and then to learn how to improve its earlier performance.
While the physical structure of Harvard's synaptic transistor has the potential to learn from history, in itself it contains no way to bias the transistor so as to properly control the SNO's memory effect. This function is carried out by an external supervisory circuit that converts the time delay between input and output into a voltage applied to the ionic liquid that either drives ions into the SNO or removes them. In response, the synaptic transistors become self-optimizing within a circuit being subjected to learning experiences.
The gain of the device adjusts over time to more efficiently provide the average performance asked of them during training. The result is that when a large network of synaptic transistors is assembled, it can learn particular responses to "sensory inputs", with those responses being learned through experience rather than directly programmed into the network.
"The transistor we've demonstrated is really an analog to the synapse in our brains," says co-lead author Jian Shi, a postdoctoral fellow at SEAS. "Each time a neuron initiates an action and another neuron reacts, the synapse between them increases the strength of its connection. And the faster the neurons spike each time, the stronger the synaptic connection. Essentially, it memorizes the action between the neurons."
The synaptic transistor could mark the beginning of a new kind of artificial intelligence: one embedded not in smart algorithms but in the very architecture of a computer. In principle, a system integrating millions of tiny synaptic transistors and neuron terminals could take parallel computing into a new era of ultra-efficient high performance.
"This kind of proof-of-concept demonstration carries that work into the 'applied' world," says research team leader Professor Shriram Ramanathan, "where you can really translate these exotic electronic properties into compelling, state-of-the-art devices." Hopefully those SOTA devices can someday be assembled into SOTA learning machines.
A paper detailing the team's findings was published last month in Nature Communications
Source: Harvard University